An Exclusive Interview with Mobileye CEO Prof. Amnon Shashua: The Future of Autonomous Driving
by Dr. Ian Cutress on January 4, 2022 11:00 AM EST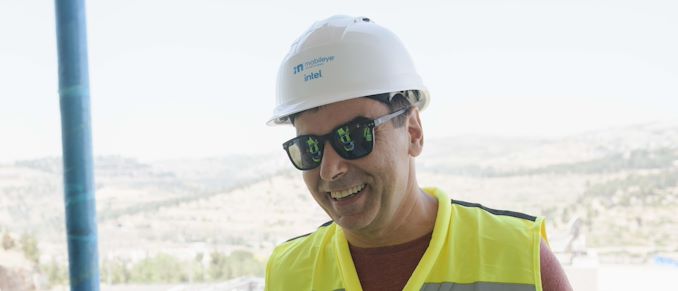
It’s hard to avoid that autonomous vehicles are a key part of how we are going to be discussing the technology and machine learning of the future. For the best part of a decade, we’ve been discussing the different levels of autonomy, from Level 1 (basic assistance) to Level 4 (full automation with some failover) and Level 5 (full automation with full failover), and what combined software and hardware solution we need to create it. One of the major companies in this space is Mobileye, acquired by Intel in 2017, and the company has recently celebrated 100 million chips sold in this space. Today we’re talking with CEO and Co-Founder Professor Amnon Shashua about the latest announcements from Mobileye at this year’s CES, including the company’s next-generation all-in-one single-chip solution for Level 4.
Professor Shashua co-founded Mobileye in 1999, covering driving assistance and autonomous driving, focusing on both system-on-chip and computer vision algorithms. The company launched its IPO in 2014 on the New York Stock Exchange, and was acquired by Intel in 2017 for $15.3 billion in the largest Israeli acquisition to date, to which he retains his CEO role and is a Senior VP of Intel. The company is set to IPO again in 2022, with Intel remaining the primary stakeholder. Prof. Shashua is still an academic, achieving his PhD from MIT in 1993 on ‘Geometry and Photometry in 3D Visual Recognition’ and being a member of faculty at the Hebrew University of Jerusalem since 1996. He currently holds the Sachs Chair in Computer Science, and has since 2007. He has published over 120 peer-reviewed papers and holds over 94 patents, some of which have evolved to form startups for using AI for the visual and hearing impaired (OrCam) or natural language creation. He is also involved in a new digital bank for Israel, the first new bank in the region for 40 years.
![]() Prof. Amnon Shashua CEO, Mobileye |
![]() Dr. Ian Cutress AnandTech |
Today’s announcement at CES 2022 focuses on the EyeQ Ultra, a new all-in-one silicon solution designed for full Level 4 autonomous driving. The goal with EyeQ Ultra is to provide a package to be introduced into robo-taxis and regular consumer vehicles in the 2025 timeframe, at a total cost of $5000-$10000 for a system-level power of around 100 W. This comes along with new advancements in algorithm development and new sensors coming directly from Mobileye/Intel partnerships, which we discuss in the interview.
Ian Cutress: You're here today because CES is a big deal for Mobileye - you're the co-founder of Mobileye, currently under the heading of Intel. For people who haven't necessarily heard of Mobileye before, can you kind of put the company into the context of where it sits today?
Amnon Shashua: We are one of the biggest providers of driving-assisted technologies using computer vision. We have a family of silicon system on chips, called the EyeQ processors, that use front-facing cameras and other sensors to power a wide spectrum of driving assist customer functions. We have shipped 100 million chips to date, and we have been on this road for 22 years. We work with almost all the major and non-major car makers, and we are also working towards full autonomous driving. This covers the entire spectrum from driving assistance, premium driving assistance, level three/level four, computer vision, driving policy, and mapping all the components that you need to build an autonomous car. In the meantime, we're also supplying to the car industry, the technology for powering driving assist today.
IC: One of the key elements to Mobileye is the high-performance low-power silicon design, paired with computer vision and autonomous driving algorithms. This week at CES, you're announcing the EyeQ Ultra – what exactly is EyeQ Ultra? I’ve read the press release, and there are some mind-boggling numbers involved.
AS: So I know, when you build a business, it's all about cost/performance metrics. So it's not just enough to have very, very good performance, you have to have very, very good cost if you want to build a viable and sustainable business. This is what Mobileye has done over the years - today on the road is our fifth-generation SoC called EyeQ5. These SOCs are based on a number of proprietary accelerators - so it's not only that you have CPUs, but you also have accelerators with a wide spectrum of workloads. Not only is it deep learning compute, but it is also multi-threaded compute, cores that are similar to when to FPGA, cores that are SIMD, VLIW, very long instruction words - a variety or diversity of accelerator cores that Mobileye over the past 16 years has been developing. Today, we have EyeQ5.
We are announcing the EyeQ6 at CES, which comes in two varieties, and the EyeQ Ultra. The EyeQ Ultra is an L4 autonomous vehicle on-chip. It has, in terms of some details, 64 accelerator cores which are divided into four families of cores. We have one family which is purely deep learning compute, we have another family which is CGRA, a coarse-grained reconfigurable array which is similar to FPGA. We have another family of cores that multi-threaded which is SIMD, VLIW. So, altogether there are 64 accelerator cores, 12 RISC-V CPU cores (each is 24 threads), and we have a GPU and a DSP by Arm. It's on a 5 nm process, and the first silicon is going to come out at the end of the fourth quarter of 2023, so two years from now. Normally in the cycle, from engineering sample to start-up project production, in all of our families of SoC, we start with a ‘cut one’ which is the first engineering sample. Then half a year later comes ‘cut two’ and then there is a PPAP (automotive supply chain) process which is very critical for making the SoC automotive grade. This takes somewhere between a half a year to a year, and then it's ready for production. So 2024 we can have vehicles with EyeQ Ultra on the road, but in terms of mass volume, the start of production automotive-grade, it's going to be in 2025. It's one package, one monolithic piece of silicon.
IC: A lot of times when we talk about silicon in autonomous driving and vehicles, there has to be backups. Is the EyeQ Ultra solution two chips for redundancy, or are you trying to plan redundancy on just a single chip? Have the rules about what customers need regarding redundancy in that respect changed over the years?
AS: So we're working at a system-level redundancy in many aspects. One level of system redundancy is about the sensing modalities that we have. We are working end-to-end using only cameras, and then end-to-end using only radars and LIDARs. So in the interest of the sensing state, the perception of perceiving the world is done through two parallel streams which do not talk to each other. The cameras do not talk with the radars and LIDARs, and the radars and LIDARs do not talk with the cameras. This gives us a system-level redundancy, we call that ‘true redundancy’. At the chip level, the 64 accelerator cores are divided into two pieces of 32 cores, creating an internal redundancy. There is an ASIL-D MCU outside of the EyeQ Ultra such that with the dual ‘ASIL-B plus ASILD’ MCU, we get an ASIL-D system. That’s at a product level – in addition, there is a fail operation, where we use an EyeQ6 Low. An EyeQ6 Low is a very, very small chip - it's about a five TOPS of silicon. It's very, very cost-efficient and that processes a number of cameras for a fail operation stream, a complete fail operation stream, such that if something goes wrong, the car can go into a safe state and stop on the side.
IC: When we see companies talking about L4 autonomous driving, they have multiple chips in play - multiple silicon pieces, whether it's multiple CPUs or multiple GPUs. It sounds like the EyeQ Ultra is designed to be an all-in-one solution, with no other silicon compute resources in play (unless it's for redundancy or failover)?
AS: We have gone through an evolution - today we have a system on the road which is just camera-based. It's not L4, but it is L2+. The first launch is in China, with the Geely Zeekr. It has 11 cameras around the car for 360 visuals and two EyeQ5 chips. The EyeQ5 is about 15 TOPS, 15 DL Tera- operations per second. So it has two such chips, and it provides an end-to-end capability. We have unedited videos on the internet showing how this car drives at multiple sites in Tel Aviv, Jerusalem, Munich, Tokyo, Paris, China, Detroit. I'm going to show this at the CES. So it's really end-to-end for L2+, but it doesn't have the robustness good enough to remove the driver out of the loop. For that, you need more compute and more sensor modalities in order to create redundancy.
So now we have another system going to go into production in early 2024. It's going to have L4 capability. It's also with the Zeekr. It is it's going to be based on six EyeQ5 chips. So it will have L4 capability with a certain limitation of ODD, the operational design domain.
We have on our robo-taxi an ECU called AVKIT 58. These are eight EyeQ5 chips, and this is powering our robo-taxi which has been debuted at IAA in Munich back in September. It's going to be on the road in the middle of 2022. and homologated by the end of 2022 in terms of the hardware to get all the permits to remove the driver from the road. The EyeQ Ultra is learning from all this evolution. So with EyeQ Ultra, after building the AV capability end-to-end, it's not only the perceptions or the driving policy or the control - you can see that from all those clips on the internet. We came to the conclusion of what is exactly the compute need that we must have in order to support a full L4, with an MTBF (mean-time between failures) high enough to remove the driver from the loop. We came to the conclusion that it is 10 times the EyeQ5, so the EyeQ Ultra is roughly a 10 x EyeQ5.
Now, why a single chip? At the end of the day, the cost matters a lot for consumers. Cost matters a lot if you want to be involved in what will evolve to be consumer autonomous vehicles. In around 2025, there will be robo-taxis, and some consumer AV. Consumer AV means you buy a car, you pay somewhere around $10,000, and you get an option by a press of the button, the car becomes level four. So you can have ‘mind off, eyes off’ where you don't need even to sit on the driver's seat. So the cost here matters a lot.
Also, the ECU with an EyeQ Ultra - the cost of that will be significantly less than $1,000. The way we designed it, the full level four system, with the cost of the ECU, the sensors, the compute, everything, in terms of cost will be significantly less than $5,000. That will enable an MSRP to the customer for around $10,000, and that's around 2025. So this is why it's very, very important to get this monolithic AV on-chip, given all the learnings that we have done, and all the evolution of multiple chips in order to understand exactly what we need in order to support a level four product.
IC: It's almost crazy to think that the major cost in design in autonomous vehicle systems in the future is going to be the sensors and not the silicon. That's how it sounds!
AS: That’s true! So with sensors, there are also some breakthroughs. So there are two types of sensors that the public is aware of - cameras and LIDARs. With LIDARs, there's a certain cost that is inherent. A LIDAR does not go down to the cost of a camera, and it will not go down to the cost of a radar. We're developing the next generation LIDAR - it's a frequency modulation coherent waves called FMCW LIDAR. I talked about it at the last CES.
But the real breakthrough in my mind will come from the next generation of radars. And I'll share this at CES, I talked about at last CES, but I'll show a project that we are building. It's called a software-defined imaging radar, it has more than 2000 virtual channels, but there are many many other important elements, not only the number of virtual channels. That enables us to develop software running on the radar output that is so high resolution, whatever we do with cameras today, we can do with this radar. So we can treat this radar as a standalone sensor in terms of building a sensing state, perceiving the world around you. So you can look at very congested traffic with lots of lots of pedestrians and vehicles - today radars have no chance in separating the different objects, stationary and moving, and pedestrians, and you can have a pedestrian near a vehicle, a vehicle under a bridge, and all sorts of distractions that could happen that radars today cannot handle. This type of radar can handle it.
Now, why is this a game-changer? Because the cost of such a radar is between a fifth to a tenth of the cost of the LIDAR. So now you can imagine a configuration where okay, we talked about the compute, you have a 360-degree of cameras, this is way below $1,000, you have a 360-degree of these imaging radars, which is also way below $1,000. You'll have a single front facing LIDAR, so front-facing which is the most critical part of the field of view. You'll have three-way redundancy, you have cameras, this imaging radar, and the LIDAR somewhere around $1,000. So you can see that all together, you're getting something which is way below $4,000 in terms of cost. And this is the key to bringing the cost level to a point in which we can have a consumer AV, because today, cost levels of self-driving systems are way above that, an order of magnitude above that, which is good enough for powering the robo-taxi, but not for a consumer level car.
IC: With the EyeQ Ultra chip that you're announcing, I think the big number that astounds me is the 176 TOPs. When we hear about other autonomous vehicle L4 driving level systems, they're an order of magnitude beyond this, so it seems amazing that you guys can claim that you're going to have an L4 equivalent system with only 176 TOPs. I know you're not necessarily going to talk about competing solutions, but I think my question to you is if TOPs the right metric in order to understand the competitiveness between different solutions?
AS: I think TOPs is not the right metric. There are actors that are trying to push TOPs as kind of the new horsepower metric, but it is very, very misleading. You know what, when people talk about TOPs, they really talk about deep learning TOPs. Even with deep learning TOPs, there are all sorts of details that are being emitted that doesn't include sparsity, it doesn't include the sparsity.
On the convolutional neural networks, there are now many new types of architectures of neural networks. Then there are many, many types of different workloads, which are not related to deep learning calculations. This is one of the strengths of Mobileye, in which, given all those years of experience of building these systems, of computer vision-based systems, and also the driving policy, we have the right combination of accelerators and CPUs to bring us to a point which is very, very efficient.
Now, it's not a new kind of a forward-looking thing. Take, for example, the Zeekr car I mentioned earlier. Already a few 1000s of vehicles have been shipped, and the functions are going to be updated over the air in the coming months, from basic driving aids to the full L2+ which provides an end-to-end capability of hands-free driving. It's not L3 or L4 yet, and you need the driver behind the steering wheel, but in terms of the capability, it's a full end-to-end hands-free driving. All of what we are showing in our unedited videos, about what this car can do, is with only 11 cameras and two EyeQ5 chips. Now it has only two EyeQ5 chips that are responsible for the entire perception of 11 cameras - this is lots and lots of compute camera processing. The cameras are 8 megapixel, so we're talking about lots and lots of data coming in to those two small EyeQ5 chips. ,But then you also have the driving policy, the planning, you know, in a self-driving system. You have sense, you have a plan, and you have to act, right? You have to do that the planning in compute. Planning is also a humongous amount of compute. If you look at competing solutions, we're able to do it on two chips, where each of them is 15 TOPs. So it tells you that TOPs is really not the right measure. You need to build a system on a chip that is sufficiently diverse, and you need to build the right algorithms that are purpose-built for the task. You have silicon vendors that are producing general-purpose chips and then they're only weak cores. To compete is try to define a new metric, which is this TOPs kind of horsepower metric. But life is much more nuanced than that.
IC: So what about TOPs per Watt? What exactly is the right power for one of these systems that we should be thinking about? Some people are still talking about 1000W sitting in the trunk!
AS: As you know, even Watts is misleading. For example, are you talking about only the deep learning engines? Are you talking about the entire SoC? Are you talking about the static power at 125 degrees? A temperature that affects so many details? When people talk about a wattage, are you talking about the system, the entire system power consumption? This is a better measure, not the chip.
Mobileye has system on chips, which are behind the windscreen. So we are talking about an automotive-grade 125 degree junction temperature behind the windscreen, and performing very, very powerful compute in systems that are way below 3 or 4 watts. That’s the system, not just the chip! So giving you a number of watts on the EyeQ Ultra doesn’t say much - I mean I can give you a number, and it's way below 100 watts. But without going into all the details about how power consumption is being measured, these numbers are also meaningless. At the end of the day, you need to measure system-level power consumption, and we're building this such that the system-level power consumption is system level, not the chip level, system level is below 100 watts.
IC: A hundred watts means we can put on a PCIe card then! That would be fun. Just to clarify, when you say 125 degrees, are you talking Fahrenheit or Celsius?
AS: Celsius.
IC: Okay, 125 Celsius because it’s automotive grade?
AS: That’s right.
IC: So that’s like Dubai, but also in the middle of the engine?
AS: Exactly!
IC: You mentioned the four types of accelerators that you have in the EyeQ Ultra - deep learning, VLIW, CGRA, and multi-threaded: can you be a bit more explicit on what these do and what partnerships you're leveraging for the IP in these?
AS: The CPUs in EyeQ Ultra are RISC-V CPUs. In the previous generations of EyeQ5, those are MIPS CPUs, and EyeQ Ultra will have 12 RISC-V CPUs. Those are IPs that we license. Our accelerators are cores that we design, and this is part of our proprietary design. Those cores have been, you know, perfected over the past 15 years. So each type of core has been silicon in previous generations, but upgraded. It's been perfected better and better and better as the technology improves, as architectures of neural networks improve, as our understanding of what kind of algorithms we need. When we talk about algorithms, it's important to make a point where you need to create internal redundancies.
So say, for example, you have an algorithm that is doing pattern recognition, detecting a car in front, and you try to perfect it as much as you can using data driven techniques. But then you want to also create another route, within the same chip, but completely different in algorithm. It could be an algorithm that takes a top view by piecing all the cameras together, and from the top view, detecting where the vehicles are. It could be an algorithm that is doing pixel-level labelling, labelling every pixel, whether it's a road or not a road. And when something is not a road, then no, it gives an indication that maybe you have either a static or a moving object. You can do pixel level segmentation for every pixel label what it is, is it a road? Is it a car? Is it a pedestrian? And so forth. So very different types of algorithms in the same chip.
We have another algorithm called VIDAR, which is taking all those 11 cameras, and creating a 3D cloud of points just like a LIDAR. So a complete Depth Map. When you have a complete depth map, you can use a completely different type of interpretation, of visual interpretation, because now you have a 3D cloud of points, and now you want to detect cars.
So every detection, every perceptual understanding that we want to make from the scene, we do it in multiple ways. This creates the need to diversify the type of algorithms and therefore the type of accelerator cores that supports those algorithms. And this is the need for those four families of accelerators. So it's not just one architecture of acceleration, it is multiple architecture, and then we have from each family, we have multiple cores, and I said the EyeQ Ultra has 64 accelerators.
IC: This chip is meant to be four or five years away. In the chip space that I usually report on, that is quite early - I know automotive have long cycles on this however. But I'll pose the same question to you that I pose to the more standard sort companies I talk to - how confident are you in predicting where the algorithms are going to be that far out, such that you're confident that what you're designing today has the right balance of compute, interconnect, memory? Also, it terms of where the algorithms are going to be in four or five years?
AS: Well this would have been a pertinent question, say five years ago! Today, we're at the point where we know exactly what we need to do in order to build a level four system, because we have been building it in smaller pieces. We’ve been building all the components, building the computer vision, and we have cars on the road with our computer vision doing end-to-end autonomous driving. We are building a separate stream of RADARs and LIDARs, and we have vehicles on the road using end-to-end autonomous driving with a safety driver now, but also autonomous driving without cameras, just relying on RADARs and LIDARs. We have been doing this for the past five years, building crowdsourcing technologies for building high-definition maps, and those maps are part of the perception right? We have done all of that, so we know exactly what the algorithms are - this is why we are designing the EyeQ Ultra today and not three years ago. We could have done that three years ago, and built a humongous chip to support L4, but then your question three years ago or five years ago would be very, very relevant. How would you know what kind of algorithms you need in order to support a L4?
Today, we know these algorithms, and this is why we know exactly what the architecture of our AV on chip, autonomous vehicle on chip, should be. We took that as building blocks of the EyeQ5, since the EyeQ5 is running now all our autonomous vehicle development. As I said, it is two EyeQ5 today, we have six EyeQ5s for L4 capability in early 2024 as a consumer vehicle in the Zeekr. It's going to be announced at CES that we have on our robo-taxi eight EyeQ5s. So we know exactly what are the algorithms, and therefore the EyeQ Ultra is respectful for all those learnings. We know exactly what are the algorithms and the architectures that we need for a level four vehicle.
IC: You said before the announcement for the Ultra chip, first silicon is in late 2023, production silicon in 2025. Can you go through where you are right now with the design? Do you have extensive RTL? It's clear that you're doing simulations? Where exactly do we stand, because you're 18 months away - which for most chips is a good chunk of the design cycle before first silicon.
AS: We are way above the 50% in RTL. So in a few months, we should have the 100% RTL and start the back-end phase. We’re working with ST Micro - our partnership with ST Microelectronics goes from 2005 From EyeQ1, to EyeQ Ultra.
IC: Other autonomous vehicles systems talk about how much they process - we've gone over the TOPs argument, but what sort of frames per second or response time is the EyeQ Ultra system targeting? Some vendors will focus on 60 frames per second, some will say 24 frames per second. What's your opinion there?
AS: Even those numbers are sometimes meaningless, because there are many many details that are being emitted. At the control level, we support between 50 to 100 hertz. In terms of perception, we're working at 18 frames per second, which is sufficient for perception. There are also all sorts of processes that are slower, some are at 9 FPS, which are not critical. They're only for redundancy, and there are processes that are on 24 FPS also. So there are many details when we talk about frames per second, it's not one monolithic number.
IC: On the discussion around chip packaging, is there anything special going on the packaging here with Ultra?
AS: So the packaging technology is ST’s IP, and they have been working on packaging for us on the EyeQ5, the EyeQ4, the EyeQ3, and EyeQ6. It's a legacy, a continuing legacy of building automotive grade packaging for very high-performance computing. All of our SoCs are, at the time of production, cutting-edge high-performance compute, and ST is matching that with the right package.
IC: In 2017, Mobileye was acquired by Intel, and you guys have been part of their financials since. We've seen the numbers for Mobileye go up and up and up over the last few quarters, so congratulations. But what exactly did the acquisition by Intel bring to Mobileye that perhaps wasn't there previously?
AS: I would say there were three elements.
One is manpower. So when we were acquired, we were at about 800 employees. Today, we are at 2500, but 800 of those 2500 are coming from Intel. So it's significant because we're not talking about individual people - we’re talking about entire teams. Building teams is difficult, so building that 800 people or team organically would have been very, very difficult.
The second is technologies. The imaging radar that I talked about is from Intel teams that have moved to Mobileye. The FMCW LIDAR development is using Intel Silicon Photonics - these are special labs of Intel, but it's also Intel teams that have been moved to Mobileye. So we're talking about technologies that are still outside of Mobileye’s organic skills. In no dream of mine could I have imagined that Mobileye would develop a LIDAR or something, we do not have that skill at all. So this is coming from Intel.
In terms of evangelizing our safety models, so back in 2017, we developed a safety model called Responsibility Sensitive Safety, RSS, which today is really the backbone of worldwide standardization of how you define the delicate line between safety and utility. As you know, the safest car is a car that doesn't move, but if you want to merge into congested traffic, you need to challenge other road users, so how you do it in a way that is from a societal point of view considered safe? So we developed that theory, made it transparent, published it, and then through Intel's policy and government groups, we have been evangelizing it. For example, there's an IEEE program called 2846. It's chaired by Intel, which is standardizing these kinds of questions. And RSS is really the starting point for asking those questions.
I think it has been a very, very successful partnership between Intel and Mobileye, and still will be. I see a fruitful partnership going forward as well.
IC: It's interesting you talk about the safety aspects, because over the past 10 years, it's been suggested that at some point, the technology will be there and we'll have L4 and L5 systems ready to go. Rather than the technology being limiting, it has been suggested that the main barrier to adoption will be that legal systems, where governments aren't ready to properly produce the laws around these types of technologies. How much thought explicitly do you put into that, into the point where you might be ahead of the legal system, ahead of the governments there?
AS: So this is exactly the question that we asked ourselves back in 2017. If we don't do something on the legal front, we will get stuck, and at that time, the common wisdom was the mileage driven [would be convincing enough]. So the more mileage you drive without intervention, which meant the safer you are, was to our mind not a sufficient metric.
When you need to put a machine on the road, it should be orders of magnitude safer than a human driver in order to be accepted from a societal point of view. How do you go and validate something like this? Validating the perceptual system is something that you can wrap your mind around and define it, but how do you go and validate the judgments that the machine makes? It needs to make judgments to merge into traffic, or if you want to change lane and the traffic is congested, so somebody else needs to slow down in order for you to merge. How do you do that in a way that is not reckless? How do you define what is reckless? How do you define what is a dangerous manoeuver? How do you define what is a safe manoeuver?
All of those are lacking in rigid definitions. A machine needs to have formal definitions - it cannot make any move just based on heuristics, because you will not be able to defend it in court later when an accident happens. So this is when we started to develop this formal theory of what it means to drive safely, what are the assumptions that people make when they drive, and how can you code it into a formal theory. Also what kind of guarantees can you give - can you give a guarantee, for example, that you'll never cause an accident? This is exactly what we set out to do. In that RSS paper, and we wrote our two papers in that project, and as I said before, it is today, really the basis of all worldwide standardization.
Today you see, for example, in Germany that there are laws that enable to remove the driver. For example, you need to homologate the design, and there are certain steps that you need to do. The UK Commission and EU Commission have legal language that gives you legal certainty for deploying an autonomous car. I think the US would also at some point have that proper language to create certainty for these kinds of technology. Israel has enacted the law as well, so it's getting there. I think 2022 and 2023, for robo-taxis, that is the correct sweet spot in terms of starting to see a deployment of autonomous ride-hailing. The learnings from it would then propel, with the right cost, in 2024 or 2025 for consumer autonomous vehicles.
IC: So on the point on robo-taxis, maybe this is particularly region-specific, but I want to posit a possible scenario to you. A tobo-taxi goes to pick somebody up, but it’s a fare that a taxi with a human driver that wouldn't necessarily pick up, either because they either look ill or they're prepared to do damage to the car. If it was a human, they would just drive off and not accept the fare, but with a robo-taxi that's not part of that situation. So at your level, are you considering these sorts of situations that could be the endpoint of where your technology is going? Or are you kind of just happy to leave that into the hands of those that are deploying it at scale?
AS: Well talking about the vandalism, there could be all sorts of things going on. So robo-taxi’s would also have a teller/operator. So the operator does not drive the car but can give instructions to the car, in case it gets stuck, in case it doesn't know what to do, and they can give it instructions. It can also solve this issue that you mentioned before, before we pick up a passenger, the operator has a view from all the cameras of the car and can make a decision of whether to pick up that passenger or not. But there are more critical issues that could come up, such as violence inside the car cabin. So the cabin is viewed from the operator's view of the cabin and can see what's going on, and can deactivate the car if necessary, or call for help. I think those issues are issues that you need to think about once you have robo-taxis at scale. I'm thinking 2022 or 2023, as robo-taxis are not yet at scale. So those issues can wait a bit, but once you really have this at scale, when you need to think about vandalism, you need to find the solutions, but I believe that those solutions will be found.
IC: On a more personal note, today I'm talking to you as CEO of Mobileye. But you are also a Professor, with the Sachs Chair of Computer Science at the Hebrew University of Jerusalem. You've co-founded other companies in the AI space, and in doing my research on you, I saw that you're co-founding Israel's first bank in 40 years, a digital bank. Do you have enough hours in the day for what you do?
AS: Well, as you know, what I do is all around artificial intelligence - everywhere where I think our AI can be transformative. Whether it is building autonomous cars and driving assist to save lives, or language models, building intelligence, general intelligence, you know, or disrupt banking as we know it. AI can help people with disabilities, whether they're blind or visually impaired, or have a hearing impairment, or are dyslexic. AI can help in all those areas, and in each such areas, I have a company for it. My sole executive position is at Mobileye, but for all those other companies I founded or co-founded, I take a chairman position, and then I guide the company. But in terms of an executive position of managing people, it's only at Mobileye.
IC: So on the machine learning front, the industry focuses a lot on computer vision, natural language, recommendation engines, and those are some of the big ones that have extended into revenue-heavy industries. But are there any areas of machine learning research you'd like to investigate deeper on, or that the industry or academia hasn't really focused on so much?
AS: Well, I think the new frontier of AI is language. Language gives you a window to intelligence, because when you have a machine that has only very, very strong pattern recognition capabilities, even say human level, or even exceeding human-level pattern recognition, you will still not say that this machine is intelligent. When you have a machine that can drive autonomously or play chess or play Go or play Minecraft, you know, exceeding human capability, you would not say this machine is intelligent. But with a machine that can master language, this is a window towards intelligence.
If you can look at what search engines have done to society - what search engines have done is that you have an encyclopedia in your pocket, right? You don't need to remember things, just simply take out your smartphone and search and you get an answer to any factual question that you have. Language AI, in language, would bring intelligence into your pocket. So you'll have access to a wise person. It's not just asking a question, but have a conversation with Albert Einstein, or have a conversation you know, with a philosopher, or have a conversation with any kind of expert that you have in mind. It will all be in your pocket. This unlocks values that are even hard to imagine today. The language frontier opens up the door towards general intelligence. Five years ago, if an AI expert talked about general intelligence, this expert would have been treated with skepticism. Today, I think it's around the corner.
Many thanks to Prof Shashua and his team for their time.
Many thanks also to Gavin Bonshor for transcription.
12 Comments
View All Comments
Unashamed_unoriginal_username_x86 - Tuesday, January 4, 2022 - link
Feels a bit unfortunate having an exclusive interview buried under CES content...Some of his answers are a bit odd to me. Handwaving algorithms improving makes some sense since it isn't required for L4, but capitalising on improvements could significantly shrink TCO (though clearly the aaS model would soften that blow.)
Ian Cutress - Tuesday, January 4, 2022 - link
L4 is basically almost complete L5, minus some safeguards for fall back.Amandtec - Saturday, January 8, 2022 - link
L4/L5 concepts are just abstractions that are probably no longer useful in this space. The press talk about them because it makes it easy to explain things but I think industry players have moved beyond this -for them it is the business metric of use case revenue vs. research cost vs. installation cost/unit * quantity.feeblegoat - Wednesday, January 5, 2022 - link
Excellent interview Ian! Really interesting times ahead for AI and for autonomous vehiclesAmandtec - Saturday, January 8, 2022 - link
This article is overdue. I have been following this space closely and was blind to the developments going on at Mobileye. Now I suspect they are going to leapfrog Tesla and Waymo two years out. Maybe I am too easily influenced, but I have seen way to many drives around town (Teslas and Waymos) that lead me to expect they are stuck in a rut with their current methods.legrosvelma - Thursday, January 13, 2022 - link
This piece has been long overdue. I have been paying close attention to this field and was completely unaware of the advances taking on at Mobileye. Now, I believe they will be able to outperform Tesla and Waymo in https://cookie-clicker2.co the next two years. Perhaps I am too easily swayed, but I have witnessed far too many Teslas and Waymos driving around town, leading me to believe they are caught in a rut with their current tactics.emmaty - Thursday, January 27, 2022 - link
It was a thorough interview. I really like the idea of growing the mobile businesses in the town. Most businesses including restaurants are prefer mobile apps to launch their menus like https://karachirestaurants.biz/zameer-ansari-gulsh... It would really benefit most of the citizens who want to deal with mobile apps.jesssie101 - Monday, February 14, 2022 - link
Very useful post. This is my first time i visit here. I found so many interesting stuff in your blog especially its discussion. Really its great article. Keep it up.<a href="https://thebigpizza.pk/">Best Pizza in Karachi</a>
jesssie101 - Monday, February 14, 2022 - link
very interesting post.this is my first time visit here.i found so mmany interesting stuff in your blog especially its discussion.. https://jewella.pk/ thanks for the post!davidtrim - Saturday, February 19, 2022 - link
The concept of autonomous is absolutely innovative yet beneficial in so many ways. One of the important factors is the reduction of stress and health risks. According to https://heartratezone.com/, many heart diseases are initiated with driving stress, an increase of traffic, and harmful vehicles pollution leads to many health risks.